Jose Robledo’s Interview
Starting date: 10.10.2022
Nationality : Argentinean
Implementing Partner : Forschungszentrum Jülich
Main supervisor: Dr. Klaus Lieutenant
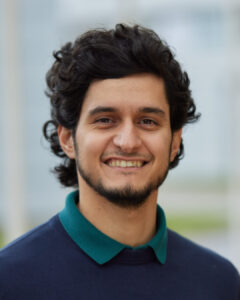
Few words about you and your research project
I’m a physicist interested in neutron related experimental data analyses.
My GNeuS project is focused on improving Small Angle Neutron Scattering (SANS) data analysis procedures by combining virtual experiments with machine learning algorithms and multivariate statistical methods. I’m currently working for the Jülich Centre for Neutron Science 2 (JCNS-2) at the Forschungszentrum Jülich (FZJ). This science research centre has a very nice campus, located in the vicinity of Jülich.
During my project I will perform two secondments, both related to Monte Carlo simulations. One will be in Denmark and will focus on porting SANS samples to GPUs to accelerate simulations on McStas, an open-source Monte Carlo code for neutron tracing. The other one will be with Nano Optics Berlin (NOB) in Berlin and will involve the simulations of a neutron reflectometer (that is to be transferred from Berlin to Budapest) in its new location at the Budapest Neutron centre (BNC). These simulations will be carried on VITESS, which is another Monte Carlo code for neutron tracing, which is hosted by FZJ.
What is your background? How have you heard about GNeuS?
I did my PhD in the National University of Córdoba and a postdoc at the Bariloche Atomic Centre, both located in Argentina. My PhD was centered in Resonant Inelastic X-Ray Scattering with synchrotron radiation. I developed a technique called Energy Dispersive Inelastic X-Ray Scattering to obtain structural information from RIXS spectra, and also designed and fabricated a spectrometer to measure these spectra in a synchrotron facility.
My first postdoc was related to Deep Inelastic Neutron Scattering in neutron time of flight spectrometers, where I developed the data reduction procedure to obtain neutron total cross sections from the transmission detectors of the VESUVIO spectrometer at the ISIS spallation facility. I have also done a master’s study on applied statistics, in which I trained convolutional neural networks to learn the classification task in sample models on neutron scattering data.
I heard about the GNeuS project by two means. The first was through the neutron source mailing list, which I strongly suggest to subscribe since there is interesting information regularly sent related to neutron science. The second was by direct recommendation of a colleague working at European Spallation Source, who saw that my profile fitted one of the proposed research projects.
Why did you apply specifically on GNeuS?
I think that the GNeuS programme is an excellent opportunity to develop myself as a researcher. This project grants many advantages, not only the possibility of investigating on a neutron topic, but also on networking, training soft skills related to research, and opportunities to participate in excellent academic courses. Throughout networking, I expect to stablish many future long-term collaborations, which will enhance my career.
GNeuS grants an excellent space for career development in neutron science.
What impacts do you expect from the GNeuS fellowship?
Since I come from another continent, one of my expectations during this fellowship is to participate in several neutron conferences around Europe. This will hopefully allow me to broaden my network of international collaborators and increase the visibility of my work. I also expect to be able to publish the results obtained during this position in relevant scientific journals.
I believe we may impact substantially in the optimization of the methodological approach in SANS data analysis. Nowadays this process is very tedious and complex for new users. There are many degrees of freedom that users have when performing the analysis, which may obscure the process and may end in the irreproducibility of the data reduction and analysis procedures. I expect to train different machine learning algorithms to assist SANS users in the data pre-processing stage (like background subtraction or model selection) in order to make it reproducible, unifying and standardizing the criterions in an optimal way.
I also want to show the potential and advantages of using machine learning algorithms in large neutron facilities, to convince the neutron community of including them as regular analysis tools.